HRTF Upsampling With a Generative Adversarial Network Using a Gnomonic Equiangular Projection
An individualised head-related transfer function (HRTF) is very important for creating realistic virtual reality (VR) and augmented reality (AR) environments. However, acoustically measuring high-quality HRTFs requires expensive equipment and an acoustic lab setting. To overcome these limitations and to make this measurement more efficient HRTF upsampling has been exploited in the past where a high-resolution HRTF is created from a low-resolution one. This paper demonstrates how generative adversarial networks (GANs) can be applied to HRTF upsampling. We propose a novel approach that transforms the HRTF data for direct use with a convolutional super-resolution generative adversarial network (SRGAN). This new approach is benchmarked against three baselines: barycentric upsampling, spherical harmonic (SH) upsampling and an HRTF selection approach. Experimental results show that the proposed method outperforms all three baselines in terms of log-spectral distortion (LSD) and localisation performance using perceptual models when the input HRTF is sparse (less than 20 measured positions).
Visit PublicationAuthors from the Audio Experience Design Team
Related Project
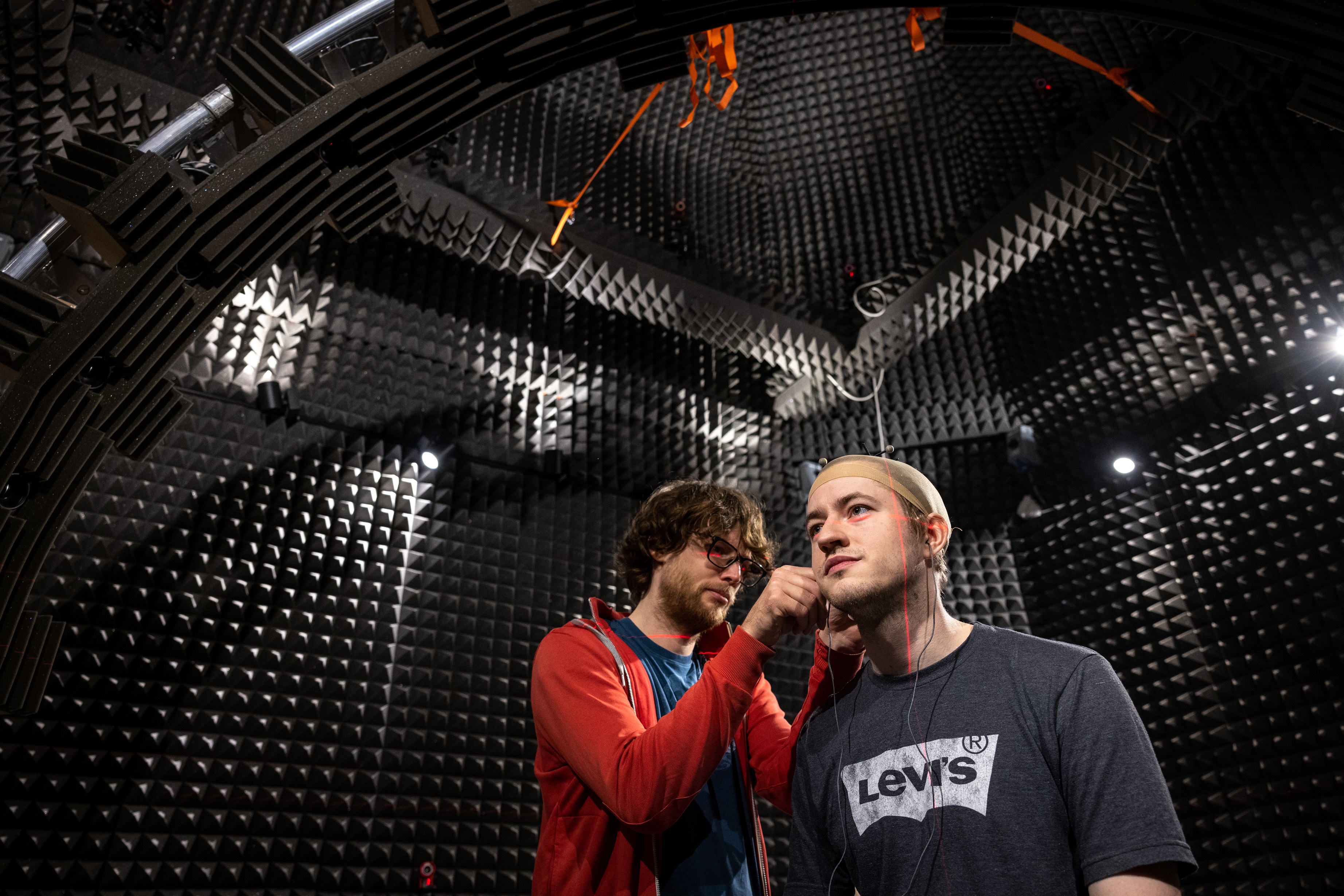
Transforming auditory-based social interaction and communication in AR/VR